
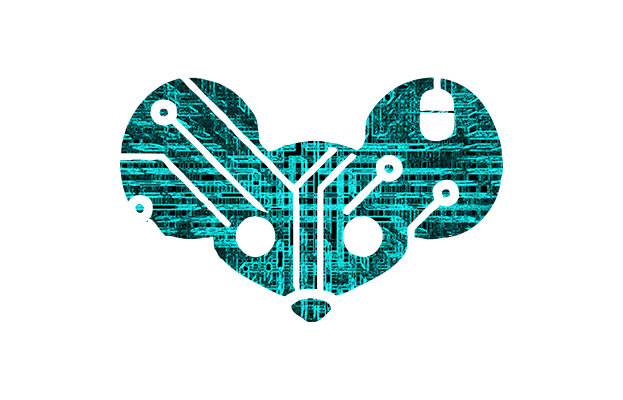
Pretty sure Trump said he wanted Zuck executed
Pretty sure Trump said he wanted Zuck executed
Same that’s why we organised between the students. Lecturers weren’t handing this out
I have been for years.
And no the choice was status quo genocide enabler and fascist genocide promoter.
My actions are aligned.
Laggy on osx
I assumed we were upvoting them to laugh at them.
From someone in the field
It lowered training costs by quite a bit. To learn from preference data (whats termed as alignment with human values), we used a very large reward model as a proxy for human feedback.
They completely got rid of this, hence also the need to have very large clusters
This has serious implications for spending though. Big companies who would have to train foundation models coz they couldnt directly use meta’s llama, can now just use deepseek.
and directly move to the human/customer alignment phase, which was already significantly cheaper than pretraining (first phase of foundation model training). With their new algorithm, even the later stage does not need huge compute
so they def got rid of a big chunk of compute by not relying on what is called a “reward” model
GRPO: group relative policy optimization
huggingface is trying to replicate their results
Not gonna watch a 30min video but the title is legit. They’ve wrote books and stuff on it, not really a secret.
https://www.vcinfodocs.com/what-is-the-network-state
edit: actually from the description/timestamps it looks like a good watch.
The right wing factions pretty much say they’ve been guaranteed as much.
Was very common in my uni. We had our full degree on mega so could read ahead.
Op needs to infiltrate the Oxford student fb group
And why can’t they wage war on the world? What are we gonna do about?
And seems Trump is laser focused on erasing Palestine and giving his donors everything they promised.
Adelson donated much more to Trump than aipac has in decades
Israel is going to annex Egypt.
Oh there’ll be a single state if Trump gets his way. But it’ll be Israel.
Because it’s an effective vote for Genocide++
Not if she is.
Correct they’re not really leftists. Tankies who consumed too much Zionist and Russian propaganda.
And yet, it got much worse almost instantly. Weird that.
No that’s a daft thing to say. Learn some nuance.
Actually the OP is outdated. They rescinded it in order to proceed with the freeze. Or at least that is what they’re claiming even though it doesn’t really even make sense.